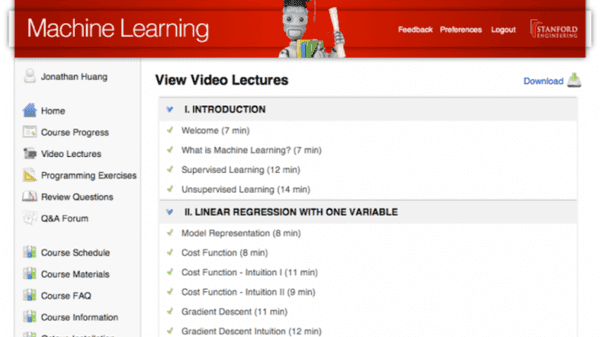
Origins of the Modern MOOC (xMOOC)
Online education has been around for decades,with many universities offering online courses to a small, limited audience.What changed in 2011 was scale and availability, when Stanford University offered three courses free to the public, each garnering signups of about 100,000 learners or more.The launch of these three courses, taught by Andrew Ng, Peter Norvig, Sebastian […]